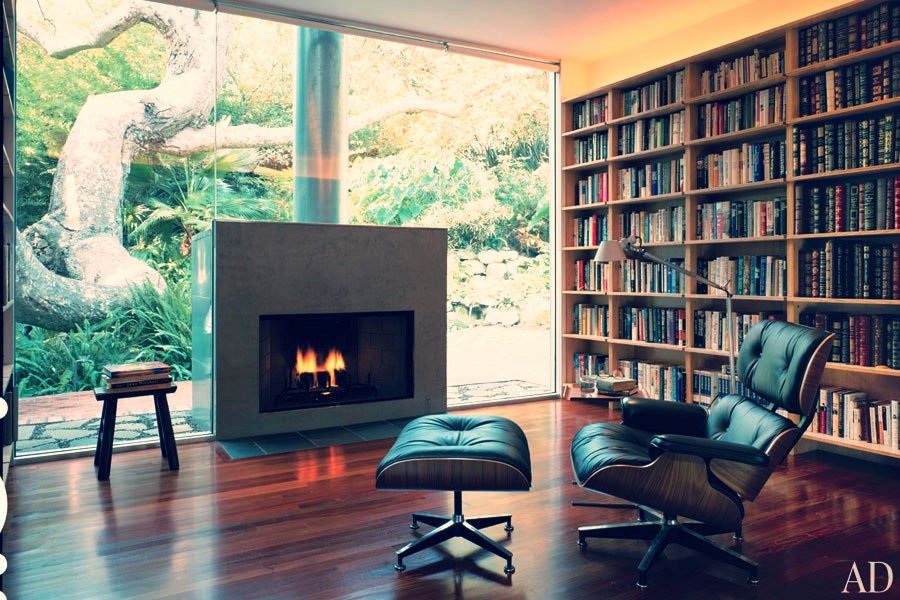
A COLLECTION OF THOUGHTS
Thoughts
&
Musings
5 Steps to Getting Started With Big Data for Small Businesses
So, you must have heard something about big data. The information you received may have made it seem as though big data is only for large corporations.
Big data is large amounts of data sourced from a business’ activities. It is not normal data hence; it cannot be analyzed so directly. It is true that big data is more suited to large companies. However, this is because big data analytics are known to be costly and time-consuming.
Thankfully, times have changed and developments in business now make it possible for everyone to benefit from big data. As a small business owner, it is essential to take part in its great benefits. This is made possible by the application of suitable tools and techniques.
Read on to learn 5 effective steps to getting started with big data for your small business.
So, you must have heard something about big data. The information you received may have made it seem as though big data is only for large corporations.
Big data is large amounts of data sourced from a business’ activities. It is not normal data hence; it cannot be analyzed so directly. It is true that big data is more suited to large companies. However, this is because big data analytics are known to be costly and time-consuming.
Thankfully, times have changed and developments in business now make it possible for everyone to benefit from big data. As a small business owner, it is essential to take part in its great benefits. This is made possible by the application of suitable tools and techniques.
Read on to learn 5 effective steps to getting started with big data for your small business.
1. Know your customers’ preferences
Data analytics starts by gathering the data acquired from customer activities also known as transactional data. With big data, you can develop highly customer oriented services because you know what they want. Start by gathering data on their experiences and behavior from any device such as laptops or phones.
2. Create a system that can identify trends
Trends in business tell you what is going on with sales, satisfaction, and so on. To benefit from big data, you must create a system that displays important information also known Key Performance Indicators (KPI’s). The system should be efficient enough to identify trends which occur in the market. A business analyst can be of great help in this area.
3. Invest in data solutions
Yes, the cost is the primary reason business owners avoid using big data, but that is hardly necessary seeing as every business requires a good investment to be successful. Invest in some data solutions to enhance your methods of acquiring, analyzing, and interpreting your data. Suitable examples for small businesses are SAS, Google Analytics, IBM Watson Analytics, and much more.
4. Know what your needs are
The tricky thing about big data is that it can provide accurate information but can also be misinterpreted. If your needs or questions for the data are not defined, the information may become useless or part of bad decisions. Take the time to review every department in your business and determine their needs. This will help you with proper analysis and interpretation. Some questions you may develop include:
Who are our best customers?
What do customers want?
What brands get the most attention and why?
5. Take action
The reason why you should use big data is to get results. Results do not come from inaction. After acquiring, analyzing, and interpreting your data, the next steps should be geared toward achievement. What do you do with the information provided by the data?
Big corporations, who use big data, take action to enjoy the following benefits:
To gain a competitive advantage by tailoring services to customer’s needs.
To make effective business decisions
To mitigate risks.
To monitor business performance
What is Big Data?
Big data is a term common to both large and small businesses. Almost every business owner has heard something about big data, but not all know what it really is.
Big data can be understood just as it sounds; it is data in large sizes. It is aptly defined as a large amount of data in both structured and unstructured forms. Big data is so named because it cannot be analyzed with traditional data processing methods or applications. It requires a different approach and software.
Companies value big data because it provides answers that increase the efficiency of a business.
Big data is a term common to both large and small businesses. Almost every business owner has heard something about big data, but not all know what it really is.
Big data can be understood just as it sounds; it is data in large sizes. It is aptly defined as a large amount of data in both structured and unstructured forms. Big data is so named because it cannot be analyzed with traditional data processing methods or applications. It requires a different approach and software.
Companies value big data because it provides answers that increase the efficiency of a business.
The concept of Big Data
Big data is characterized by three 3Vs. Each part explains the concept of big data and how it is relevant to your business.
Volume- Volume is the most important aspect of big data definition. It is sourced from various aspects of a company. The volume of big data can be hundreds of petabytes about business transactions.
Variety- Variety describes the unlimited forms of data. Data can be in structured or unstructured forms such as numbers, text, and so on.
Velocity- Data can be received in split seconds. It can also be received in long hours. The velocity of data simply refers to the speed with which it is received and analyzed.
The use of Big Data
Not every business or company can use big data. Smaller businesses can easily perform data analytics with traditional software applications. Big data stands out because its uses are also highly significant. Here are some important uses of big data.
Customer Satisfaction
In any organization, customer satisfaction is vital. Big data is used to gather accurate information on customer needs and preferences from surveys, social media, calls, and so on.
Product Development
Product development is not to be taken lightly in any business. It is important to anticipate consumers’ demands to avoid loss and rejection. Big data analytics helps companies to predict the response of the consumers to a new or modified product.
Efficiency in Operations
The availability and proper analysis of data in an organization helpto improve efficiency in operations. This is the most significant influence of big data on companies. Efficient mode of operations guarantees customer satisfaction and sustainability.
Challenges of Big Data
Getting to know about big data goes beyond its definition; it also involves knowing what challenges lie in using or receiving big data. Despite its invaluable uses,the issues faced with big data have been existent for a long time. Companies still struggle to keep the paceand find effective solutions to them.
Storage
Big data increases steadily with almost no way to control it. Organizations have always had to search for valid ways to store big data because it cannot be discarded.
Time and analysis
Big data is BIG. This means more time, effort, and different methods will be applied in analyzing the data. While this cannot be helped, it is a standard issue because time is important to an efficient organization.
What to Consider When Hiring a Data Science Team
Organizations do not face identical challenges when using data to gain insights to better run their operations. In previous blogs, we have identified possible challenges that organizations face and professionals that can be hired to help overcome these hurdles. We also discussed the roles that those professionals can play in the organization.
When looking to hire a data science team, it is important that all hiring decisions be based on the need to solve practical problems. In this post, we will shift attention to discussing factors that need to be considered when looking to hire a data science team.
In this article, we will discuss what to consider when hiring a data engineer, a data analyst, a business intelligence developer, and a data scientist. Keep in mind that for a successful data-driven organization emphasis must be placed on developing capable teams rather than individuals. A variety of background and experiences bring improved efficiency to the team. Interaction and learning from each other should be promoted within the team to interpret data well and develop the best recommendations. dc Analyst can help you build a team that fits your goals and can help you achieve your vision.
Organizations do not face identical challenges when using data to gain insights to better run their operations. In previous blogs, we have identified possible challenges that organizations face and professionals that can be hired to help overcome these hurdles. We also discussed the roles that those professionals can play in the organization.
When looking to hire a data science team, it is important that all hiring decisions be based on the need to solve practical problems. In this post, we will shift attention to discussing factors that need to be considered when looking to hire a data science team.
In this article, we will discuss what to consider when hiring a data engineer, a data analyst, a business intelligence developer, and a data scientist. Keep in mind that for a successful data-driven organization emphasis must be placed on developing capable teams rather than individuals. A variety of background and experiences bring improved efficiency to the team. Interaction and learning from each other should be promoted within the team to interpret data well and develop the best recommendations. dc Analyst can help you build a team that fits your goals and can help you achieve your vision.
Data Engineer
Data engineers are also referred to as data architects or ETL developers. Their main role is to import different data sources into a single repository. The data engineer is responsible for organizing data that will be relied on by the data science team. When hiring a data engineer, there are specific interpersonal, technical, and work experience qualities you need to consider.
Team Collaboration
The data engineer should be able to work with other team members without unnecessary competition.
Communication Skills
The data engineer will need to identify data that can meet needs of decision makers and understand business rules that need to be applied to the data. This information will be received from business leaders and IT staff. A data engineer needs to be adept at interviewing people to gather the necessary information to make projects efficient.
Real World Experience
The data engineer needs evident knowledge and work experience of data extraction, transformation, and loading. Knowledge of a popular data ETL tool coupled with a technical certification is essential with when hiring a data scientist.
Professional Knowledge
Work experience and a technical certification in relational databases are essential. Every organization is different. Determine the best relational database platform for your organization to decide on which relational database and ETL tool knowledge is required for your engineer.
Basic Engineer Qualifications
Knowledge and a technical certification of Hadoop and NoSQL databases are essential. Within the Hadoop ecosystem, it is important to ensure the data engineer is well versed in data movement tools.
Business Intelligence (BI) Developer
The BI developer is tasked with identifying reporting needs of decision makers. The person in this role is uniquely qualified to translate reports and dashboards to enable generation of reports without IT assistance. We refer to this as self-service reporting. When hiring a BI developer you need to look for the following:
Team Work
Proficient in Business Operations
Analytical Thinking
The BI developer will work with decision makers in identifying their reporting needs. The BI developer should have a good understanding of how analytics is used in decision making.
Good Communication
The BI developer needs good interviewing skills to enable gathering of reporting needs
Data Visualization
The BI developer should be able to design reports and dashboards that effectively communicate data to the entire team.
Working Knowledge of SQL
The BI developer needs a good understanding of SQL to create queries to provide required reports.
Knowledge, working experience, and a technical certification of a BI tool is essential. Commercial and open source tools are available. Thus, you must determine what is best for your organization.
Data Analyst
A data analyst is responsible for statistical analysis of data. When hiring one you need to look for the following:
Training
Training in quantitative techniques at the appropriate level is essential. Depending on the organization training could be required at the bachelor, masters or Ph.D. level.
Communication
The data analyst will be communicating technical information to non-technical people so they should be able to present information in a simple way. They may also need to train others and write reports. Good speaking and writing skills are therefore essential.
Proficient in Business Operation
The analysts should have a basic and advanced data analysis skills depending on your organization’s needs. Knowledge of statistical software such as IBM SPSS, SAS, R, Stata, and Minitab among others. Your organization needs to identify which statistical tool will meet its needs.
Data Scientist
A data scientist can apply advanced tools and techniques to understand patterns that exist in data. When hiring a data scientist you need to look for the following:
Excellent Communication
Data science is a very technical area, so a data scientist should be able to communicate technical results to non-technical business people. Communication skills are critical because data scientists work in collaboration with business people in identifying problems. A clear understanding of the business problem and how data can be used is important.
Creative
A data scientist needs to be creative in identifying data to be used and in handling data inadequacies.
Good Computer Programming Skills
Skills in data science languages such as R and Python are essential. A deep understanding is not necessary, but the data scientist should be able to solve data science tasks.
Adequate Quantitative Skills
A strong background in statistics and machine learning is essential. The data scientist should be able to correctly identify and use models in problem-solving.
Professional Knowledge
Working knowledge of database design and SQL queries is important. This will enable the data scientist to acquire relevant data for their analysis. A basic understanding of Hadoop tools for big data analysis is especially important.
To identify people with relevant skills organizations need to use multiple interviewing approaches. It is easy to identify technical skills with practical sessions but other skills such as communication and creativity may be challenging to find. Use of hypothetical situations can be used to gauge how a candidate would handle a practical situation. A portfolio of their previously completed project should also be factored in when hiring.
The dc Analyst team is always ready to help you build a data science team that makes sense for your organization. Contact us to get started!
6 Indicators You Need a Data Science Team
In our digital economy every organization generates a sizable amount of data. There is real value in understanding and acting on insights and solutions that lay within this data. To be successful at gathering insights from data an organization needs a team of experts with various skill sets to complement each other and work collectively towards a common objective of getting value from the organization's data.
All organizations are not equal. The volume and variety of data differs, therefore, each organization has its unique challenges. The types of challenges faced dictate the type of experts that you need to consider bringing on board.
In our digital economy every organization generates a sizable amount of data. There is real value in understanding and acting on insights and solutions that lay within this data. To be successful at gathering insights from data an organization needs a team of experts with various skill sets to complement each other and work collectively towards a common objective of getting value from the organization's data.
All organizations are not equal. The volume and variety of data differs, therefore, each organization has its unique challenges. The types of challenges faced dictate the type of experts that you need to consider bringing on board.
If you find that your organization is facing these challenges you may need to hire a dc Analyst data science team to help simplify your needs:
Receiving multiple data using various sources and team members
Your IT or supervisory team is creating company performance reports
Your marketing and sales teams is in need of statistical analysis for campaigns
Your company is struggling to wrangle and organize your ever growing database
In this article we will discuss the different challenges organizations face and data analysts experts that often help organizations overcome those challenges.
Multiple Data Sources
At the most basic level data analysis is done using spreadsheets and various reports provided by varying team members. This approach has several shortcomings. First there is no standardized way of importing the data and applying necessary transformations on the data according to the organization’s business rules and objectives.
With every person doing data analysis on areas they feel is important the key performance indicators are difficult to identify. Secondly, due to the first shortcoming different people doing analysis on the same business processes are likely to arrive at different conclusions. This confusion wastes valuable time as it is lost investigating where differences come from instead of using data to collectively improve business operations. Thirdly, creation of multiple copies of data from various sources is to reconcile in the process of investigating and wrangling data.
When such problems arise within an organization it is time to bring in an data analyst expert who is skilled at integrating multiple data sources into a single repository using business rules. The single repository then becomes the common data source that is relied upon for information across the organization for data analysis and reporting.
Data analyst with the ability to gather, organize, and present data are often referred as data architects, data engineers, or ETL developers. These experts have an important role of ensuring data quality and consistency.
Relying on IT to Create Reports
When your organization constantly relies on your IT team to create business reports an unacceptable load is placed on the IT team. Valuable time is also lost waiting for reports to be gathered and presented. IT teams have a distinct role within your organization that involves the maintenance and planning for your technology needs.
When reports are created by your IT team they may fall short of what is required by your business team. To avoid a lack of information consider asking a business intelligence (BI) developer to handle some of your data processing needs.
A BI developer acts as a liaison between your business team and your reporting needs. They are uniquely experienced in helping you understand their reporting needs. BI developers create reports and dashboards that can be used by your business team to meet their needs without relying on IT. The reports can also be scheduled to run at specified intervals of time and automatically sent to those who need them. This is referred to as self-service reporting.
Need for Statistical Data Analysis
Marketing. If your organization needs statistical analysis on market research data, experimental data, or data stored in a warehouse a data analyst should join your team. Data analysts help design surveys and systems that can help you understand your customers. Information data analysts can draw inferences from data to help you understand your customer preferences and buying habits. They also prepare reports that effectively communicate results of statistical analyses in simple and easy-to-understand presentations.
Manufacturing. Data analysts support engineers and scientists with information they gain from their investigations. They interpret data to enable scientific and manufacturing efforts. For example, a data analyst will help an engineer design an experiment to identify optimal manufacturing conditions. Another example is a data analyst partnering with a medical investigator to conduct a clinical trial of a new drug and obtaining market approval.
In addition, data analyst help organizations implement data driven quality improvement programs like 6 sigma. Armed with such information your business is able to optimize business processes. In many cases, data analysts can also train team members on how to analyze and interpret data.
Unable to Cope with Data Growth
In every organization there are data growth projections and measures devised to cope with growth in data volume. When the systems in place can no longer handle new data volumes it is time to bring in experts skilled in application of big data technologies. Signs of inability to handle growth in data volumes include reports taking too long to run, spending a lot of time tuning queries, and trying to split analytical databases.
When existing systems cannot handle new types of data it is important to implement an alternative system to ensure your data is accurate and usable. Data analysts are able to leverage technologies such as Hadoop and NoSQL databases to ensure analytical operations continue.
Predictive Analytics Are Required
If your organization realizes the need for deeper analytics beyond reporting than bringing in a data science expert is the recommended next step. A data scientist is able to pose the right questions that have business value, use data to get answers, and effectively communicate to decision makers.
In many cases organizations can use predictive insights to capture relationships that exist within their data. Examples of such needs include: predicting buying behavior from demographic data and purchase history, segmenting customers into different groups, and recommending products based on the findings. Data scientists apply predictive models on the data infrastructure created by a data engineers to gain insight from the data and communicate such insights to decision makers.
Integrating Analytics with Products
If your organization needs analytic insights to be integrated into a product then your software developer who will work closely with a data scientist. For example, a data scientist develops a predictive model that recommends products that were bought by similar customers. The data scientist and the software developer will work closely to sure the recommendation engine is properly implemented in the shopping cart. Another example of a software engineer and a data scientist working together is when a credit company uses a predictive model to score clients. Or an application for credit managers is developed to help them quickly score customers.
Determining if you need a data analyst or data science team requires a practical look at the way your organization is operating. Pay attention to these high level indicators as well as consult a dc Analyst team member to learn more about how your company can benefit from gathering, organizing, and interpreting your data.
How to Analyze Data
After your team and data analyst have finished setting your objectives and gathering data you need to analyze your data to meet your objectives. When analyzing data you can use descriptive, visual, inferential, or modeling techniques. In this article we discuss various data analysis techniques and tools to use in analyzing your data.
Summarizing Data Using Descriptive Statistics
Descriptive statistics help you summarize and understand your data. There are different techniques for summarizing your data depending on if your data is categorical or continuous. Categorical data refers to observations that fall into distinct categories for example male or female. Continuous data refers to observations that do not have any distinct categories such as weight.
After your team and data analyst have finished setting your objectives and gathering data you need to analyze your data to meet your objectives. When analyzing data you can use descriptive, visual, inferential, or modeling techniques. In this article we discuss various data analysis techniques and tools to use in analyzing your data.
Summarizing Data Using Descriptive Statistics
Descriptive statistics help you summarize and understand your data. There are different techniques for summarizing your data depending on if your data is categorical or continuous. Categorical data refers to observations that fall into distinct categories for example male or female. Continuous data refers to observations that do not have any distinct categories such as weight.
When your data is categorical the most useful descriptive technique to use is count. You count the number of observations that occur in each category. For example, when you have one variable such as gender you count the number of people who are male and those who are female. When you would like to know the number of people in each category as a proportion of the total you use a percentage. In the gender example we can calculate the percentage of those who are male and the percentage of those who are female.
As you summarize categorical data you are not limited to one variable. To summarize into categorical variables we use a cross tabulation. In a cross tabulation one variable forms the rows and the other variable forms categories. We then count the number of observations that fall in each category. If in our example we also have an education variable we would be interested in knowing the education levels of males and females. These education variables could be defined categories: no education, primary, secondary, college and university.
For continuous variables there are descriptive measures that tell us how our observations cluster around a single value and those that tell us how our observations are spread. The mean and the median are two common measures that are used to summarize data. The mean is an appropriate measure when we have observations almost falling on either side. The median is an appropriate summary when we have most observations falling on one side such as our observations are skewed.
If we collect observations on weight of adult patients we can use the mean to get the typical weight of a patient. If we collect observations on salaries we will have a few people earning much more than others, in that case the median would be a better summary.
The minimum, the maximum, the range, and the standard deviation tell us how observations are spread. The minimum tells us the lowest observation, the maximum tells us the highest observation, and the range gives us the difference between the lowest and the highest observation in our data. The variance and the standard deviation tell us how a mean value varies.
The confidence interval is calculated from the standard deviation and it gives us the upper and lower bounds of a mean value. When you have two continuous variables a correlation coefficient helps you understand the strength and direction of relationship.
A negative coefficient shows you when one variable increases the other variable decreases. A positive coefficient shows you when one variable increases the other variable decreases. A correlation value close to zero shows you there is weak or no relationship. A value of 0.5 shows moderate strength while a value close to 1 shows you there is a strong relationship.
Visualizing Data With Graphs
There are different tools for visualizing categorical and continuous data. To visualize categorical data you use a pie chart or a bar chart. A pie chart divides a circular shape into angular portions that enable you to see the count or percentage of observations that are in each category. A pie chart can only be used to visualize one categorical variable. A bar chart helps you visualize categorical data using vertical or horizontal bars that show you the count or percentage of observations in each category.
You can add the count or percentage of each category on the bars for easy comparison. Bars that are taller than the others show more observations in those categories. A bar chart can be used to summarize one or two categorical variables.
To visualize continuous observations you can use a histogram, a box plot, a scatter plot or a line plot. A histogram uses bars similar to a bar chart to visualize continuous observations. The key difference is that bars in a bar plot are for a single category while bars in a histogram show a range of values. A box plot summarizes data using a box and whiskers. The whiskers on both ends of the box plot show you the minimum and maximum observations in your data. Observations that lie beyond the whiskers are outliers.
The box shows you where half of your observations lie and within the box there is a line that shows you where the median lies. The histogram and box plot are useful for visualizing the distribution of your observations. The scatterplot helps you visualize the relationship between two continuous variables. It helps you visualize the direction and strength numerically shown by a correlation coefficient.
Making Inferences From Data
The techniques we have discussed so far help you summarize your data. To test hypotheses about your data you use inferential techniques. There are different techniques for continuous and categorical variables.
A Chi-square test helps you test if there is any relationship between categorical variables. For example, in summarizing categorical data example we can use a Chi-square to test if education levels of men and women differ. For continuous variables we are mostly interested in the mean, where we can use T tests or analysis of variance (ANOVA).
There are three variants of the T test that help us test if the mean of one variable differs from a target mean, if the means of two variables differ and if the mean of one variable differs at two different time points. ANOVA extends T tests by helping us test if more than two means are different.
To help support the process of data analysis your data analysts will use both commercial and open source tools have been developed. Popular commercial data analysis tools include IBM SPSS, SAS, Stata, Excel, and Minitab. These tools provide a graphical user interface and a programming language for data analysis. R is a popular open source tool that is used to analyze data by writing programs. All of the tools and techniques we have mentioned support all the data analysis techniques we have discussed.
How to Gather Data
When you need to make informed decisions you need to rely on accurate data provided by a data analyst. To gather accurate data you must begin by collecting, analyzing, and interpreting the right data. In an order to collect the accurate data you need to follow an organized and systematic way of gathering all the pieces of information from the sources available to you. When gathering data you can collect quantitative data, qualitative data, or both.
Quantitative data are observations that are expressed in numbers and you can meaningfully summarize using statistical techniques. For example, the number of visitors to a website is qualitative data. Qualitative data provides you with descriptions and you cannot summarize it meaningfully with statistics. For example, if you ask your customers why they decided to purchase your product rather than your competitors, you will get qualitative data. In this article we discuss how to gather data from Google Analytics, Adobe Analytics, social media, and point of sale transactions.
When you need to make informed decisions you need to rely on accurate data provided by a data analyst. To gather accurate data you must begin by collecting, analyzing, and interpreting the right data. In an order to collect the accurate data you need to follow an organized and systematic way of gathering all the pieces of information from the sources available to you. When gathering data you can collect quantitative data, qualitative data, or both.
Quantitative data are observations that are expressed in numbers and you can meaningfully summarize using statistical techniques. For example, the number of visitors to a website is qualitative data. Qualitative data provides you with descriptions and you cannot summarize it meaningfully with statistics. For example, if you ask your customers why they decided to purchase your product rather than your competitors, you will get qualitative data. In this article we discuss how to gather data from Google Analytics, Adobe Analytics, social media, and point of sale transactions.
Preparing to Gather Data
Before you begin collecting data there are several preliminary preparations and best practices provided by leading data analysts.
Identify the issue to be addressed by collecting data and set your objectives.
Make a decision on the approach and methods you will use to gather data. Decide on who or what you will collect data about, your groups of interest, and geographical areas from where you will the collect data.
Make a decision on type of data you will collect. You can collect quantitative, qualitative, or both.
Identify your sources of data. You can decide to collect your data from one or multiple sources. Make sure your data sources help you meet the objectives you set.
Estimate the period you will take to collect your data.
Types of Data
There are various forms of data available to collect, organize, track, and analyze data. Today the most essential data is captured online and observes visitor behaviors, locations, and other general information. We’ve compiled a list of the most popular data gathering platforms and services, as well as provide insights into the best ways to benefit from the information they provide.
Google Analytics Data
Google Analytics (GA) is a service provided by Google to assist marketing and website development teams with understanding the traffic patterns and behaviors of visitors. GA helps you collect data on total visits, traffic sources, bounce rate, and ecommerce goals. This data helps you measure return on investment and build conversion strategies that are feasible and slightly proven, which may reduce risk.
Before you can begin collecting data via GA you need to add a tracking code on your website pages. The tracking code is a piece of JavaScript code that you place within the head tag of your page. Another way you can add a tracking code to your pages is by using Google Tag Manager (GTM). With GTM you avoid the problem of modifying code.
Using a tracking code is the classical way of gathering data but Google is encouraging users to migrate to Universal Analytics (UA). UA is just an updated version of GA that gives you more features.
By default GA gathers data on the web page, browser, user location, and language but this is not the only data you can collect. Using JavaScript code or GTM you are able to customize the data that will be collected. By customizing the data you collect, you only gather data relevant to your website so you are able to create reports that are useful to your business.
The best way to harness the power of GA or UA is to partner with your data analyst to determine the most useful data to your business.
Adobe Analytics Data
Adobe Analytics is a web analytics solution that works in a similar way to GA. To collect data on Adobe Analytics you need to add JavaScript code in a similar way to GA. Adobe Analytics provides Dynamic Tag Management to help you manage your tags without any need for coding skills.
Although GA and Adobe analytics are similar they have their differences. GA offers a free and a paid version while Adobe Analytics only offers a paid version of its analytics platform. GA stores your data for up to 25 months while Adobe Analytics stores your data for as long as you are a customer.
Adobe Analytics enables you to collect data from websites, email, mobile devices, client-server devices and most devices connected to the internet. The tracking code that facilitates data collection can be placed on the client device or on the server side. Many data and business analyst find this information very useful and concise.
When deciding whether to place your tracking codes on server or client side consider the following issues:
When JavaScript is disabled on a browser it may not be possible to collect data.
Device limitations of inability to run JavaScript may prevent gathering data.
Sensitive data needs to be protected so that it is not viewable on the browser.
Social Media Data
Social media data is information we gather from social networks that tells us how users are viewing, sharing, and engaging with our content and profiles. Some of the social media data that we can gather are number of shares, likes, mentions, followers, and comments. From our social media data we are able to calculate key performance indicators (KPI) that tell us how the brand is performing. Facebook pages give you KPIs on engagement, likes, impressions, and other valuable metrics.
To successfully use social media data the first step is to identify the goal that will be achieved by using social media data. For example, you can target to improve customer service by analyzing user sentiment. Another thing you need to be aware about social media data is its limitations. For example, some social networks are skewed in terms of gender or age of their users. Any data collected from such networks will therefore not be representative of an actual situation. Once you have identified KPIs that are important to your business goals you can rely on tools available in the market to help you gather data.
Point of Sale Data (POS)
Data collected at a POS can be identifying or non-identifying. Identifying data includes details like names, email, physical address, and phone number. Identifying information is very useful because you can use it to link data existing in other systems. Non identifying information like if a customer is a parent; is also useful to the business and may be easier to acquire.
Personal data is stored in a POS system together with products or services purchased. The collected data is then moved to a purpose built system like a data warehouse or a CRM from where it can be sourced to make informed decisions.
When you are moving data from your POS system to your purpose built system there are three important aspects to consider:
Make sure the data you are moving is of good quality. Completeness, accuracy, and validity are the three quality aspects you need to look out for.
Update data as often as possible. Daily updates are good but an even shorter update interval is better, such as every 12 hours.
Make sure the purpose built system where you move data has the capacity for analysis and extraction of actionable information from data.
By collecting, analyzing and acting on data from multiple sources you are able to have a complete understanding of your customers. With a good understanding of customers you are able to personalize your offerings. As you sit down to determine the type of data you need remember to consult with your data or business analyst to ensure the data you are gathering is relevant and useful in achieving your overall objectives.
How to Use Data Analysts for Your Business
Information investigators, commonly known as data analysts, play key roles in a range of tasks that involve gathering, arranging, and interpreting measurable data. The nature of a data analyst in Washington, D.C. varies from business to business and project to project. For example, a hospital data analyst concentrates on performing financial analysis of the hospital’s operations, physician, and ancillary rates. While an audit analyst works to ensure compliance and maintain litigation files that track progress and results.
Value of Data and Information
Data analysis or information examination is vital to businesses because it involves developing methods of assigning numerical values to various business operations. Many analysts are uniquely qualified to recognize efficiencies, identify waste, and recommend conceivable upgrades to policies and operations. Indeed, no business survives without breaking down accessible information, and compiling data that gives them legs to remain competitive in their industry and market.
Information investigators, commonly known as data analysts, play key roles in a range of tasks that involve gathering, arranging, and interpreting measurable data. The nature of a data analyst in Washington, D.C. varies from business to business and project to project. For example, a hospital data analyst concentrates on performing financial analysis of the hospital’s operations, physician, and ancillary rates. While an audit analyst works to ensure compliance and maintain litigation files that track progress and results.
Value of Data and Information
Data analysis or information examination is vital to businesses because it involves developing methods of assigning numerical values to various business operations. Many analysts are uniquely qualified to recognize efficiencies, identify waste, and recommend conceivable upgrades to policies and operations. Indeed, no business survives without breaking down accessible information, and compiling data that gives them legs to remain competitive in their industry and market.
The application and analysis of data is broad. An organization may need to introduce new variations of its current line of natural juice. A data analyst in Washington D.C. could be charged with compiling relevant factors, and provide avenues on the best way to launch the new products as well as identify key markets. Likewise, a sales executive of a manufacturing company realizes that there is a major inefficiency in a division’s supply chain. A data analyst is capable of identify where the inefficiency begins and recommend the most profitable and cost effective ways to improve efficiency and reduce waste.
Whether you need to make key decisions on your next marketing campaign, launch a new project, or improve your everyday business operations, data analysis is an effective way to solve many key issues and challenges your business is facing. Through data you may be able to answer some of your most perplexing operational questions such as the percentage of clients that are most likely to give you repeat business. Or develop a target persona for your next big product launch.
Role of Data Analysts Today
Simply analyzing information is not adequate from the perspective of settling any decision or forward business strategy. How you translate and implement analyzed information is also vital. In most cases, data analysis provides a basic decision making framework, however, an experienced data analyst in Washington, D.C., also develops a supporting and simplified implementation and overview summary. This report often organizes discoveries, breaks a large scale profile into more easily digested material, and identifies important insights from the dataset to equip your team with the most essential information needed.
Data analysts simplify and interpret numbers into plain English. Every business gathers information, from statistical surveying to transaction figures to transportation logistics to suppliers. Data analysts gathers this information and uses it to help organizations improve operations, reduce waste, and better serve clients and customers.
It is also important to differentiate the role of data analyst and business analyst. The terms data analyst and business analyst are frequently utilized interchangeably. At larger organizations a data analysts plays a key role in setting the future strategies of the company. If employed by a smaller scaled business a data analyst or business analyst in Washington, D.C. often provide similar services. However, business analysts employed by larger organizations focus more on the everyday operations and procedures of a business and how to improve efficiency and cost.
How Data Analysis Works
Most data and information is stored in a digital database or framework and accessed via a device or computer. Data analysts gather information in a variety of ways including visiting the site of the project, independent research, and an in depth look at client files, transactions, and customer accounts. Information is often compiled on location or from a remote office. Most analyst work traditional office hours, however, depending on the project and time frames an analyst may be required or requested to work a weekend or on call.
Data Collection. A standout amongst the most essential things any data analyst does is gathering, sorting, and studying distinctive arrangements of information. Their core focus is nailing down a settled overview of the information. This overview is often surveyed and observed over time and during planning and development stages.
A supermarket may request that a data analyst gather the hours that specific representatives work alongside net revenues for certain days, weeks, or even hours to determine the team’s profitability. An ecommerce store might need to identify hard numbers on where visitors are originating from, the amount they are spending on buys, and whether bargains like free shipping have any bearing on overall earnings for the business.
These are a few distinct ways a data analysts is employed by businesses looking for insights and answers into questions that affect sales and expansion. The information is often controlled, standardized, and adjusted for implementation. Data analysts ordinarily utilize PC frameworks and complex count applications to get their numbers nailed down, yet there is still a ton of scholarly know-how that goes into making these frameworks work.
Extrapolation and Interpretation. Experienced data analysts often develop summaries of what the data implies and shares relevant insights with responsible parties within the business or organization. Obtaining hard numbers on deals figures for a given Christmas season, for instance, is to some degree helpful all by itself; however, it is typically most profitable when these figures are stacked against numbers from earlier years or different seasons as a state of correlation.
Business analyst in Washington, D.C. are often approached to assists entrepreneurs and businesses with contrasts in numbers from year to year or from location to location. Data analysts more often than not have the aptitude to identify measurable qualities of things, as well as clarify what they mean.
Projections and Advisory. Data and business analysts are usually charged with advising leaders and management on how certain information can be used to change or enhance operations. These improvements and recommendations are necessary when considering rolling out improvements and other changes. An example is a hospital that is looking to improve patient release time. A data analyst may observe operational patterns, insurance billings, and types of procedures to determine possible cause of delays and how to address them.
Is A Data Analyst Right for Your Business?
The majority of businesses and organizations can benefit greatly from the insights and expertise of a data analyst in Washington, D.C. Information investigation plays a vital role is identifying areas of improvement in operations, marketing, and efficiency. Analysts in the fields of advertising, sales, and logistics utilize data to discover market white space, optimize supply chains, and improve customer retention.
Through the proper application of information your business can understand customer patterns, eliminate downtime, and maximize time. Whether launching a product, determining the best methods for shipping, or identifying the best location for your next store; a data analyst can provide the information you need to make the most informed decisions based on various factors. These factors can include demographics, community statistics, and relevant competitor analysis.
Determining the role of data analyst in your company is sometimes a challenge. It is always recommended that you meet with a data or business analyst to gain practical insights into how your business may utilize data and information to remain competitive in your marketspace.