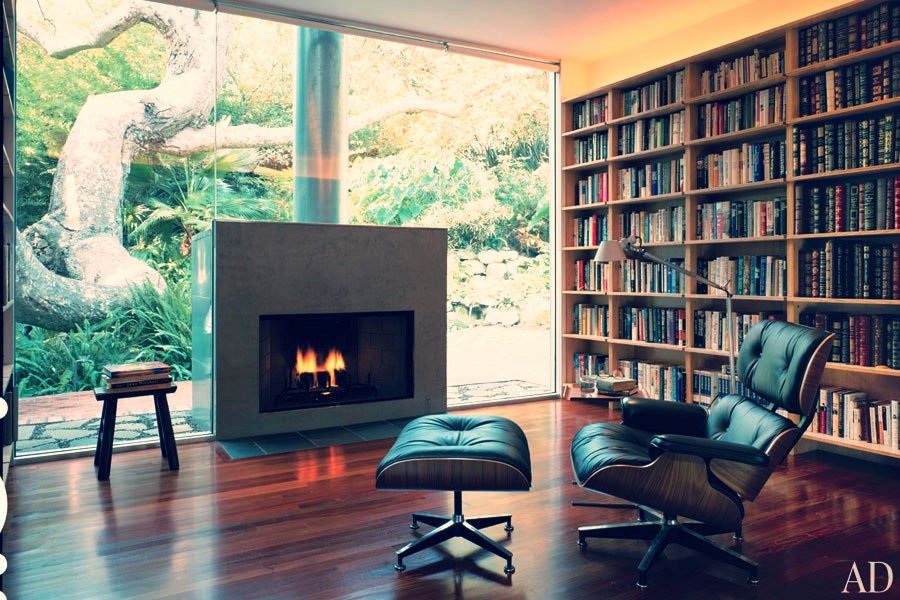
A COLLECTION OF THOUGHTS
Thoughts
&
Musings
6 Indicators You Need a Data Science Team
In our digital economy every organization generates a sizable amount of data. There is real value in understanding and acting on insights and solutions that lay within this data. To be successful at gathering insights from data an organization needs a team of experts with various skill sets to complement each other and work collectively towards a common objective of getting value from the organization's data.
All organizations are not equal. The volume and variety of data differs, therefore, each organization has its unique challenges. The types of challenges faced dictate the type of experts that you need to consider bringing on board.
In our digital economy every organization generates a sizable amount of data. There is real value in understanding and acting on insights and solutions that lay within this data. To be successful at gathering insights from data an organization needs a team of experts with various skill sets to complement each other and work collectively towards a common objective of getting value from the organization's data.
All organizations are not equal. The volume and variety of data differs, therefore, each organization has its unique challenges. The types of challenges faced dictate the type of experts that you need to consider bringing on board.
If you find that your organization is facing these challenges you may need to hire a dc Analyst data science team to help simplify your needs:
Receiving multiple data using various sources and team members
Your IT or supervisory team is creating company performance reports
Your marketing and sales teams is in need of statistical analysis for campaigns
Your company is struggling to wrangle and organize your ever growing database
In this article we will discuss the different challenges organizations face and data analysts experts that often help organizations overcome those challenges.
Multiple Data Sources
At the most basic level data analysis is done using spreadsheets and various reports provided by varying team members. This approach has several shortcomings. First there is no standardized way of importing the data and applying necessary transformations on the data according to the organization’s business rules and objectives.
With every person doing data analysis on areas they feel is important the key performance indicators are difficult to identify. Secondly, due to the first shortcoming different people doing analysis on the same business processes are likely to arrive at different conclusions. This confusion wastes valuable time as it is lost investigating where differences come from instead of using data to collectively improve business operations. Thirdly, creation of multiple copies of data from various sources is to reconcile in the process of investigating and wrangling data.
When such problems arise within an organization it is time to bring in an data analyst expert who is skilled at integrating multiple data sources into a single repository using business rules. The single repository then becomes the common data source that is relied upon for information across the organization for data analysis and reporting.
Data analyst with the ability to gather, organize, and present data are often referred as data architects, data engineers, or ETL developers. These experts have an important role of ensuring data quality and consistency.
Relying on IT to Create Reports
When your organization constantly relies on your IT team to create business reports an unacceptable load is placed on the IT team. Valuable time is also lost waiting for reports to be gathered and presented. IT teams have a distinct role within your organization that involves the maintenance and planning for your technology needs.
When reports are created by your IT team they may fall short of what is required by your business team. To avoid a lack of information consider asking a business intelligence (BI) developer to handle some of your data processing needs.
A BI developer acts as a liaison between your business team and your reporting needs. They are uniquely experienced in helping you understand their reporting needs. BI developers create reports and dashboards that can be used by your business team to meet their needs without relying on IT. The reports can also be scheduled to run at specified intervals of time and automatically sent to those who need them. This is referred to as self-service reporting.
Need for Statistical Data Analysis
Marketing. If your organization needs statistical analysis on market research data, experimental data, or data stored in a warehouse a data analyst should join your team. Data analysts help design surveys and systems that can help you understand your customers. Information data analysts can draw inferences from data to help you understand your customer preferences and buying habits. They also prepare reports that effectively communicate results of statistical analyses in simple and easy-to-understand presentations.
Manufacturing. Data analysts support engineers and scientists with information they gain from their investigations. They interpret data to enable scientific and manufacturing efforts. For example, a data analyst will help an engineer design an experiment to identify optimal manufacturing conditions. Another example is a data analyst partnering with a medical investigator to conduct a clinical trial of a new drug and obtaining market approval.
In addition, data analyst help organizations implement data driven quality improvement programs like 6 sigma. Armed with such information your business is able to optimize business processes. In many cases, data analysts can also train team members on how to analyze and interpret data.
Unable to Cope with Data Growth
In every organization there are data growth projections and measures devised to cope with growth in data volume. When the systems in place can no longer handle new data volumes it is time to bring in experts skilled in application of big data technologies. Signs of inability to handle growth in data volumes include reports taking too long to run, spending a lot of time tuning queries, and trying to split analytical databases.
When existing systems cannot handle new types of data it is important to implement an alternative system to ensure your data is accurate and usable. Data analysts are able to leverage technologies such as Hadoop and NoSQL databases to ensure analytical operations continue.
Predictive Analytics Are Required
If your organization realizes the need for deeper analytics beyond reporting than bringing in a data science expert is the recommended next step. A data scientist is able to pose the right questions that have business value, use data to get answers, and effectively communicate to decision makers.
In many cases organizations can use predictive insights to capture relationships that exist within their data. Examples of such needs include: predicting buying behavior from demographic data and purchase history, segmenting customers into different groups, and recommending products based on the findings. Data scientists apply predictive models on the data infrastructure created by a data engineers to gain insight from the data and communicate such insights to decision makers.
Integrating Analytics with Products
If your organization needs analytic insights to be integrated into a product then your software developer who will work closely with a data scientist. For example, a data scientist develops a predictive model that recommends products that were bought by similar customers. The data scientist and the software developer will work closely to sure the recommendation engine is properly implemented in the shopping cart. Another example of a software engineer and a data scientist working together is when a credit company uses a predictive model to score clients. Or an application for credit managers is developed to help them quickly score customers.
Determining if you need a data analyst or data science team requires a practical look at the way your organization is operating. Pay attention to these high level indicators as well as consult a dc Analyst team member to learn more about how your company can benefit from gathering, organizing, and interpreting your data.
How to Organize and Wrangle Data
Your data analyst in Washington D.C. often begins each project with organizing data. Organizing your data makes it very easy to gather relevant information from your data. In an organization there is often multiple sources of data that need to be brought together to provide a complete view of your processes.
The process of combining data from multiple sources into a single repository is referred to as data integration. For example an organization that sells products online needs to organize data on sales, store inventory, items returned by customers, orders placed from suppliers, and revenue for each product. Through data integration all of this data is combined and segmented to provide valuable insights.
Your data analyst in Washington D.C. often begins each project with organizing data. Organizing your data makes it very easy to gather relevant information from your data. In an organization there is often multiple sources of data that need to be brought together to provide a complete view of your processes.
The process of combining data from multiple sources into a single repository is referred to as data integration. For example an organization that sells products online needs to organize data on sales, store inventory, items returned by customers, orders placed from suppliers, and revenue for each product. Through data integration all of this data is combined and segmented to provide valuable insights.
Understanding Data Integration
Data integration is one of the most important pillars of data organization. By performing data integration you are able to remove duplicates in your data, correct errors in your data, apply transformations on your data according to business rules, and store your data. When you have very small volumes of data you are often able to manually move them from different sources into a common store. However, large volumes of data manual integration is not viable so you need to rely on a dedicated data integration tool.
Data organization requires a strategy and designed approach that is able to cope with the speed at which data arrives, its various structures, and volume. Data is often categorized as structured, semi-structured, or unstructured. Structured data is neatly organized into rows and columns. Semi-structured data has some data in rows and columns and others that cannot be organized into rows and columns. Unstructured data does not have any notion of rows and columns.
Data volume often ranges from a few kilobytes to terabytes. Data also has the ability arrive at intervals of milliseconds, minutes, hours, days or even weeks. Depending on your data volume, structure, and frequency at which data arrives you need to select an appropriate tool.
Each organization has different data management challenges that cannot be solved with a single tool. Identifying the right tool or tools is a prerequisite for gaining value from your data. In this post we are going to look at three tools that you can use to organize and analyze your data: Excel, databases, and Hadoop. We will discuss situations in which each is appropriate.
Excel
Excel is widely used for data organization and analysis because it is easily available and very user friendly. Excel is an excellent tool for organizing structured data with volumes that are able to fit within your system memory. With Excel you are able to easily perform calculations using formulas built in functions or custom built functions. With PowerQuery you are able to extract data from different sources into Excel without much coding required. Excel also enables you to import data from the web from databases such as a SQL Server and Mysql. Importing and integrating data from files such as .csv, .xml, text files, Azure, and Excel data tables and other data sources is also simplified.
Once your data is in Excel you may begin analyzing it with other compatible tools in the Excel software family, known as add-ons. For example, with PowerPivot you are able to analyze your data using pivot tables and pivot charts. With PowerView you are able to visualize your data using charts and maps.
Databases
Excel helps you organize and analyze structured data that fits within your system memory. When your data cannot fit within your system memory or it is not structured databases are the right tools. Databases are able to handle gigabytes or terabytes of data. They are often broadly categorized as relational (SQL) databases and NoSQL databases. Examples of relational databases are Oracle, SQL Server, Mysql, IBM DB2, and PostgreSQL. Examples of NoSQL databases are MongoDB, Cassandra, and HBase.
Relational databases are suitable for organizing large volumes of data that are structured. They have a language referred to as SQL which is used to manipulate the gathered data. With relational databases you are able to import data from other relational databases and business applications like CRM and user friendly files such as .csv, text as well as other mainframe databases and legacy applications.
To integrate data from different sources into your relational database you can use SQL or rely on a dedicated data integration tool. Data integration tools help your data analysts source your data, clean your data, and load your data into your database. Once your data has been cleaned and stored in your relational databases you can use business intelligence applications for data analysis and visualization. Examples of business intelligence applications are IBM Cognos, Tableau, and Qlikview.
Additionally, NoSQL databases are suitable for organizing very large volumes of data that are stored on multiple servers and are semi-structured or unstructured. NoSQL databases are an excellent choice when relational databases cannot handle the frequency, volume, and variety of data that needs to be organized and analyzed.
Hadoop
Hadoop is an ecosystem of data management tools that have been developed by Apache to handle growth in data because existing tools could not handle the volume, variety and velocity of data. Often data of such magnitude is difficult to host on a single server. Therefore Hadoop was developed as a system that is able to process data stored on hundreds or even thousands of servers and it has been a success.
The software is designed to handle very large amounts of data; whether the data is structured or unstructured. With Hadoop you are able to process data whether it continuously streams in or arrives in batches. It has a specialized file system referred to as Hadoop file system (HDFS) for storing data.
Hadoop has the tools to import data into HDFS and export data out of HDFS. The data movement tools enable your data analyst to get data from different sources such as databases, social media, web, and files. Add-ons such as Sqoop and Pig enable you to source data and move it into Hadoop. Sqoop specializes in exporting data from relational databases to Hadoop. Pig enables you to source structured and unstructured data and import it into Hadoop. Once your data is in Hadoop you are able to write MapReduce programs in Java to analyze your data.
As data analysis continues to evolve more and more tools are introduced to support data gathering, structuring, and presenting. For example, due to the complexity of writing MapReduce code various tools have been developed to simplify data analysis in the platform. Hadoop Hive enables you to develop data warehouses and analyze data using a similar language to SQL. Or to discover patterns in your data you can use Spark to develop machine learning algorithms. To manage structured and semi-structured data within Hadoop you use Hbase. These are some of the tools you can use to organize and analyze your data when captured by Hadoop, that simplify the process.
Selecting the right tool to organize and analyze your data is very important in understanding your data. With the right tool you are able to reap the benefits of insights within your data and thus help you reduce waste, improve customer retention, and develop reliable business strategies. Armed with knowledge from your data you are able to make more informed brand decisions.